FURI |
CUR Decomposition Method for Dimensionality Reduction of High Dimensional Alloy Data
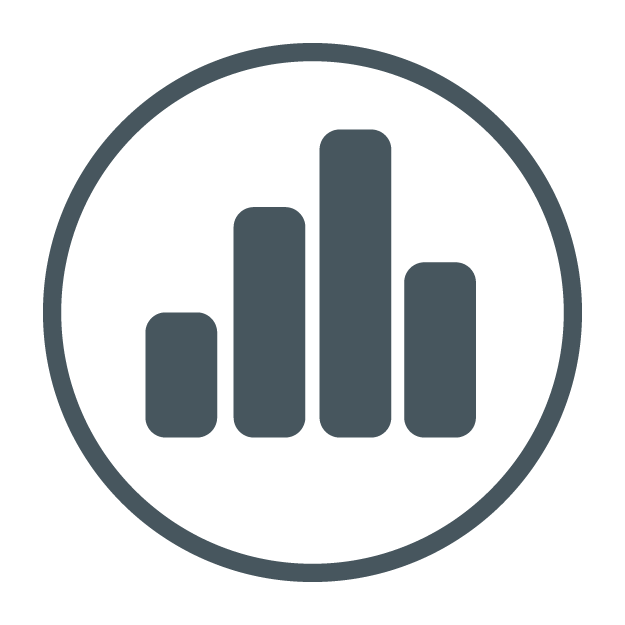
High Entropy Alloys have potential for application in structural and aerospace industries due to their desirable material properties. These alloys consist of five or more elements, leading to a large search space for material prediction machine learning models, and highly dimensional empirical datasets. For chemical datasets, it is difficult for models to interpret the output of embedded feature selection methods, but CUR decomposition, a low-rank dimensionality reduction method, has shown success in this domain. This project will assess ability of CUR decomposition as a dimensionality reduction method, for high-dimensional alloy data, before training a support vector regression classification model.
Student researcher
Charles Benjamin Klafter
Mechanical engineering
Hometown: Phoenix, Arizona, United States
Graduation date: Spring 2022